Beyond Object Detection: Unveiling the World with Pixel-Perfect Precision - The Power of Instance Segmentation
Instance segmentation is the pixel-level labeling of individual objects in an image, combining object detection and semantic segmentation for detailed image understanding.
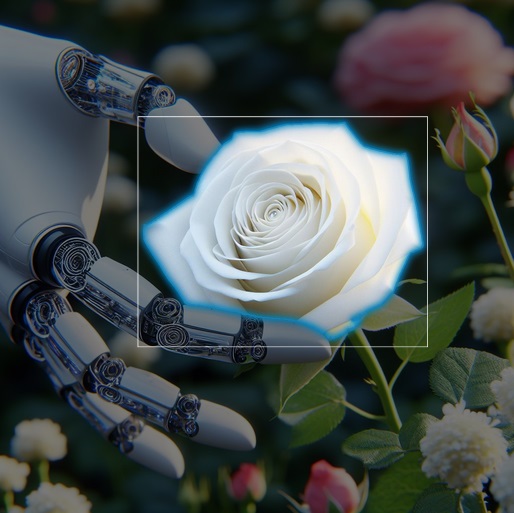
In the realm of computer vision, instance segmentation emerges as a sophisticated technique for dissecting an image and understanding its content on a granular object level. It delves deeper than simply identifying the presence of objects, venturing into the intricate task of meticulously outlining the boundaries of each individual object and assigning a distinct label to it. This empowers the computer to not only recognize a cat in a picture but also differentiate between multiple cats, pinpointing their exact locations and providing a clear separation between them.
Imagine a photograph bustling with activity, containing a group of friends gathered in a park. Instance segmentation would meticulously analyze this scene, recognizing each person as a separate entity. It wouldn’t just provide bounding boxes encasing the entire group, but rather, it would trace the outline of each individual, capturing the specific pose and silhouette of every friend. Additionally, it would assign a unique identifier to each person, enabling us to distinguish between them, even if they are standing close together. This fine-grained analysis proves invaluable in various applications where precise object identification and distinction are paramount.
Instance segmentation, an advanced computer vision method, has transformed the analysis and interaction with visual data. It goes beyond mere object identification, intricately delineating precise boundaries at the pixel level. This nuanced approach provides a deeper comprehension of visual scenes compared to conventional methods, opening doors to a plethora of applications across various industries. Its granular detail renders it indispensable across numerous sectors, marking it as an invaluable asset in modern technological landscapes.
Instance segmentation and semantic segmentation both analyze images, but with key differences in their level of detail. Imagine a picture with several cats. Semantic segmentation would classify all the furry regions as “cat,” but wouldn’t distinguish between individual felines. Instance segmentation, on the other hand, would not only identify each cat but also meticulously outline their fur boundaries, allowing you to count the exact number of cats and potentially even track their movements.
One prominent field benefiting from instance segmentation is autonomous vehicles. By accurately segmenting individual objects like pedestrians, vehicles, and traffic signs, self-driving cars can gain a more nuanced understanding of their surroundings. This precise object identification allows them to navigate complex environments safely, differentiating between a stopped car and a cardboard box on the road, for instance. Instance segmentation also plays a crucial role in traffic monitoring systems, enabling real-time analysis of traffic flow and efficient management of transportation networks.
Beyond the realm of transportation, instance segmentation is transforming the medical field. In medical imaging analysis, it empowers doctors to precisely identify and segment individual cells, tissues, or organs within an X-ray, MRI, or CT scan. This pixel-level accuracy facilitates earlier and more accurate diagnoses, particularly in the detection of cancerous tumors or abnormalities. Furthermore, instance segmentation can be used to track the progress of treatment over time, allowing for more personalized and effective healthcare strategies.
In the field of manufacturing and robotics, instance segmentation plays a vital role in automating tasks and ensuring quality control. By segmenting individual objects on a production line, robots can be programmed to perform precise actions like grasping specific parts or identifying defective products. This level of automation streamlines manufacturing processes, improves efficiency, and minimizes errors. Additionally, instance segmentation can be employed in visual inspection systems, where it helps detect anomalies in product quality, leading to enhanced production consistency.
The applications of instance segmentation extend far beyond the examples mentioned above. In the agricultural sector, it can be used to assess crop health by segmenting and analyzing individual plants. In retail, it can be employed to track customer behavior by identifying and counting people within a store. These are just a few examples of how instance segmentation’s ability to provide a granular understanding of visual data is fostering innovation and progress across a wide range of industries.
Instance segmentation is a rapidly evolving field within computer vision, constantly being refined and improved. As machine learning algorithms become more adept at pattern recognition and image analysis, we can expect even more sophisticated applications to emerge. This technology holds immense potential in various sectors, from automating tasks in manufacturing and robotics to enhancing the user experience in augmented reality applications. FasterLabeling, expediting the annotation process for training instance segmentation models, proves invaluable in enhancing efficiency. By reducing the time and resources required for manual annotation, FasterLabeling streamlines the creation of high-quality training datasets, ultimately leading to faster development and deployment of instance segmentation solutions. With its ability to provide an in-depth understanding of visual data at the object level, instance segmentation, aided by FasterLabeling, is poised to play a transformative role in shaping the future of computer vision and its many real-world applications.